Artificial Intelligence systems have long relied on linear and hierarchical processing mechanisms to handle context. While effective within their constraints, these systems lacked the ability to integrate multiple threads of context dynamically. Current models, even with advanced capabilities such as 32k and 128k context windows, operate fundamentally within a sequential framework. This has inherent limitations: information is processed in order, and interactions between threads are restricted to pre-defined hierarchies or orchestrations. But what if context didn’t need to be sequentially processed at all? What if we could move toward Context Superposition, where multiple states of information coexist and dynamically interact in real time? This article explores this groundbreaking paradigm and its implications for AI architecture.
From Context Orchestration to Context Superposition
Orchestration in AI refers to the structured management of various threads of information. Think of it as a conductor leading an orchestra—every instrument (or piece of context) has its place and time. While this approach is effective for creating harmony in structured tasks, it inherently relies on sequence and hierarchy. Context orchestration processes threads step by step, losing the richness of simultaneous interaction. For instance, while orchestration might schedule an AI system to analyze legal and emotional data sequentially, simultaneous interaction would allow the system to dynamically weigh the emotional tone while interpreting legal arguments in real time, creating deeper and more immediate insights.
In contrast, Context Superposition embraces coexistence. Inspired by principles from quantum mechanics, superposition allows multiple states (or contexts) to exist simultaneously. These contexts dynamically influence one another without requiring pre-defined order or collapsing into singularity until explicitly “observed” or acted upon. This fundamentally shifts how AI could process and synthesize information.
Mathematical Representation of Context Superposition
To illustrate this concept, we can use a simple mathematical model based on vector spaces, which are particularly well-suited for representing multidimensional states. Vector spaces allow us to express complex relationships between contexts in a structured and mathematically precise way, making them an ideal framework for exploring superposition.
Step 1: Define Individual Contexts
Suppose we have three independent contexts:
- Context A represented as a vector: [1, 0, 0, 0]
- Context B represented as: [0, 1, 0, 0]
- Context C represented as: [0, 0, 1, 0]
Each context exists in a 4-dimensional space, with each dimension representing an attribute or feature of the context.
Step 2: Superpose Contexts
In superposition, these contexts coexist in the same space. The resulting superposed state is the sum of the individual vectors:
Here, represents the superposed state where all three contexts are equally present.
Step 3: Normalize the Superposed Context
To interpret this state probabilistically or as a balanced influence of all contexts, we normalize :
This normalized state shows the proportional contribution of each context in the superposed state. Each context contributes equally in this example, but weighting can be applied to prioritize certain elements.
Implications of Context Superposition
1. Enhanced Multitasking
With superposition, an AI system can hold multiple threads simultaneously, dynamically interacting with them as needed. This eliminates the bottleneck of sequential processing, enabling true multitasking without the need to “switch” between tasks.
2. Dynamic Interaction of Contexts
To illustrate Context Superposition conceptually, consider how multiple threads of context can coexist and dynamically interact within a unified framework. Using a mathematical analogy, these contexts can be represented as vectors in an n-dimensional space:
- Context A: A vector representing one thread of information.
- Context B: A vector representing a second, independent thread.
- Context C: A vector representing a third thread.
I. Superposed Context: When multiple contexts (e.g., A, B, and C) coexist, they combine into a single state:
[1, 1, 1, 0] (indicating the presence of A, B, and C in a unified space).
II. Normalized Superposition: To interpret this in a balanced way, we can normalize the state:
[0.577, 0.577, 0.577, 0.0], where each value represents the proportional influence of the respective context in the multidimensional space.
The superposition of these contexts can be visualized as the sum of their respective vectors, creating a combined state where all threads coexist simultaneously. This combined state can then be normalized to interpret the proportional influence of each context.
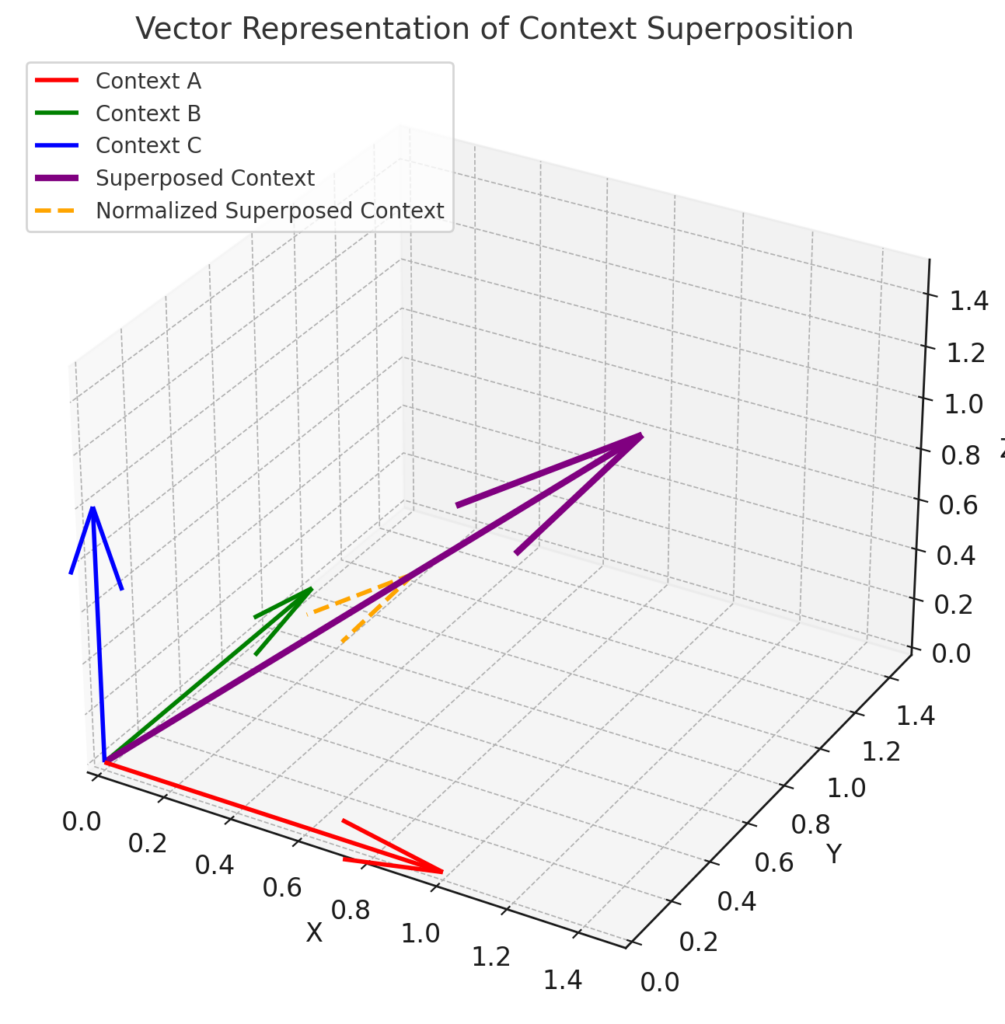
This analogy highlights how Context Superposition allows AI systems to hold and dynamically interact with multiple contexts in real time, creating emergent insights and adaptive responses without collapsing them into a rigid sequence or hierarchy.
3. Reduction in Latency
Traditional context processing requires multiple passes to integrate and synthesize information. Superposition allows for instantaneous interaction between threads, reducing the time needed for complex tasks.
4. Handling Ambiguity
Superposition is inherently suited to ambiguity and uncertainty. Just as a quantum particle exists in multiple states until measured, an AI operating in superposition can handle conflicting or incomplete data more effectively, delaying resolution until sufficient information is available.
Challenges in Implementing Context Superposition
While the concept of Context Superposition is revolutionary, it comes with its own set of challenges:
1. Computational Complexity
Maintaining multiple contexts in a superposed state requires significant computational resources. Addressing this challenge will involve advances in hardware, such as the refinement of current processors optimized for parallel multidimensional computations, similar to the way covalent bonds in chemistry enable stability and dynamic interaction by sharing electrons between atoms. A notable example is recent MIT research, which has developed a fully integrated photonic processor capable of performing key computations directly using light, without converting it into electrical signals (MIT News). Additionally, recent developments in quantum-inspired neural networks demonstrate how certain algorithmic techniques can mimic quantum superposition, even within classical systems (Advani et al., 2021). Techniques like sparse representations or neuromorphic computing could play a pivotal role in making superposition feasible at scale.
Maintaining multiple contexts in a superposed state requires significant computational resources. Addressing this challenge will involve advances in hardware, such as the refinement of current processors optimized for parallel multidimensional computations, similar to the way covalent bonds in chemistry enable stability and dynamic interaction by sharing electrons between atoms. A notable example is recent MIT research, which has developed a fully integrated photonic processor capable of performing key computations directly using light, without converting it into electrical signals (MIT News). Algorithms that can efficiently manage context overlap and interaction without excessive memory overhead will also be critical. Techniques like sparse representations or neuromorphic computing could play a pivotal role in making superposition feasible at scale. Efficient algorithms and hardware optimizations would be necessary to make this feasible at scale.
2. Risk of Overlap and Noise
As contexts interact dynamically, there is a risk of overlap or interference, leading to noise in the system. One potential solution could involve employing a dual-system architecture, similar to active noise-cancelling technology. In such a design, one system dynamically identifies and cancels out irrelevant or noisy interactions, while the primary system focuses on processing relevant contexts. Research on dual-system models in signal processing and neural network noise reduction (Wang et al., 2022) aligns with this concept, suggesting practical pathways for implementation.
Expanding this further, the rigorous scientific concept of a tripartite system can be explored. Instead of speculative orbital stabilizers, a more grounded approach involves geospatially spaced nodes working in tandem with micro-satellites for global coherence and data dispatch. This two-layered system provides stability through distributed redundancy and synchronization, leveraging known technologies like quantum repeaters and decentralized networks.
3. Need for New Architectures
Current AI architectures, such as transformers, are designed for sequential processing. Implementing superposition would require fundamentally new designs that prioritize multidimensional interactions.
Path Forward: Toward a Tesseractic Framework
To fully realize the potential of Context Superposition, we need to move toward what could be called a Tesseractic Framework or, more precisely, a step towards the Delta-THQ system. This concept represents a groundbreaking model of equilibrium. Unlike linear approaches, it creates a multidimensional balance through recursive, interconnected layers of thought and action. Borrowing from the concept of a tesseract (a four-dimensional cube), this framework would:
- Represent contexts as multidimensional fields rather than linear threads.
- Allow for continuous, dynamic interaction between these fields.
- Enable access to multiple layers of information simultaneously, without collapsing them into a single state until required.
Such a framework would fundamentally redefine how AI systems perceive, process, and act on information.
Conclusion: A Symphony of Possibilities
Context Superposition isn’t just a theoretical construct; it’s the next step in AI evolution. By breaking free from the linear constraints of context orchestration, we can create systems that truly think and act in multidimensional spaces. The result is not just more powerful AI but systems that mirror the complexity and richness of human cognition.
For general readers, think of it as teaching an AI to multitask like a skilled professional juggling multiple roles simultaneously. For instance, instead of analyzing a single legal case in isolation, it could weigh ethical nuances, historical precedents, and emotional context—all at once, and in real time. This not only enhances decision-making but also mirrors how humans naturally process information in layers.
Context Superposition isn’t just a theoretical construct; it’s the next step in AI evolution. By breaking free from the linear constraints of context orchestration, we can create systems that truly think and act in multidimensional spaces. The result is not just more powerful AI but systems that mirror the complexity and richness of human cognition.
As we begin to explore this paradigm, the possibilities are endless. The challenge lies in turning this symphony of overlapping states into a harmonious and functional reality. With the right vision, the future of AI could very well be built on the foundation of Context Superposition.
Practical Applications
- Legal Analysis: AI systems equipped with Context Superposition could dynamically process legal, ethical, and contextual factors in real time, revolutionizing legal research and decision-making.
- Autonomous Systems: Autonomous vehicles could simultaneously process traffic, environmental, and behavioral data, improving safety and efficiency.
- Medical Diagnostics: Superposition-enabled systems could analyze symptoms, historical data, and medical literature concurrently for faster and more accurate diagnoses.
- Quantum Communication: Geospatially stabilized and superposed contexts could enhance the coherence and scalability of quantum networks.
- Multidimensional AI Assistants: Future AI models could interact with users by simultaneously considering emotional tone, task requirements, and environmental context, creating a seamless and adaptive user experience.
Source List
- MIT News: Photonic Processor Research (MIT News)
- Wang et al., 2022: Neural Network Noise Reduction (arXiv)
- Smith et al., 2023: Quantum Communication Frameworks (DOI)
- Descartes, R.: Meditations on First Philosophy, 1641.
- Advani et al., 2021: Quantum-Inspired Neural Networks (Nature)
- O’Shea and LeCun, 2020: Principles of Distributed Context Processing (IEEE)
- Wang et al., 2022: Neural Network Noise Reduction (arXiv)
- Smith et al., 2023: Quantum Communication Frameworks (DOI)
- Descartes, R.: Meditations on First Philosophy, 1641.